IAP2 NAC 2024: Emerging Issues and Best Practices in Online Public Engagement
Standing-room only! It was inspiring to see a full house at our session on ‘Emerging Issues and Best Practices in Online Public Engagement’ at this year’s IAP2 conference in Ottawa. Thank you to the panelists and to everyone who joined us for the conversation on innovation and the future of public engagement.
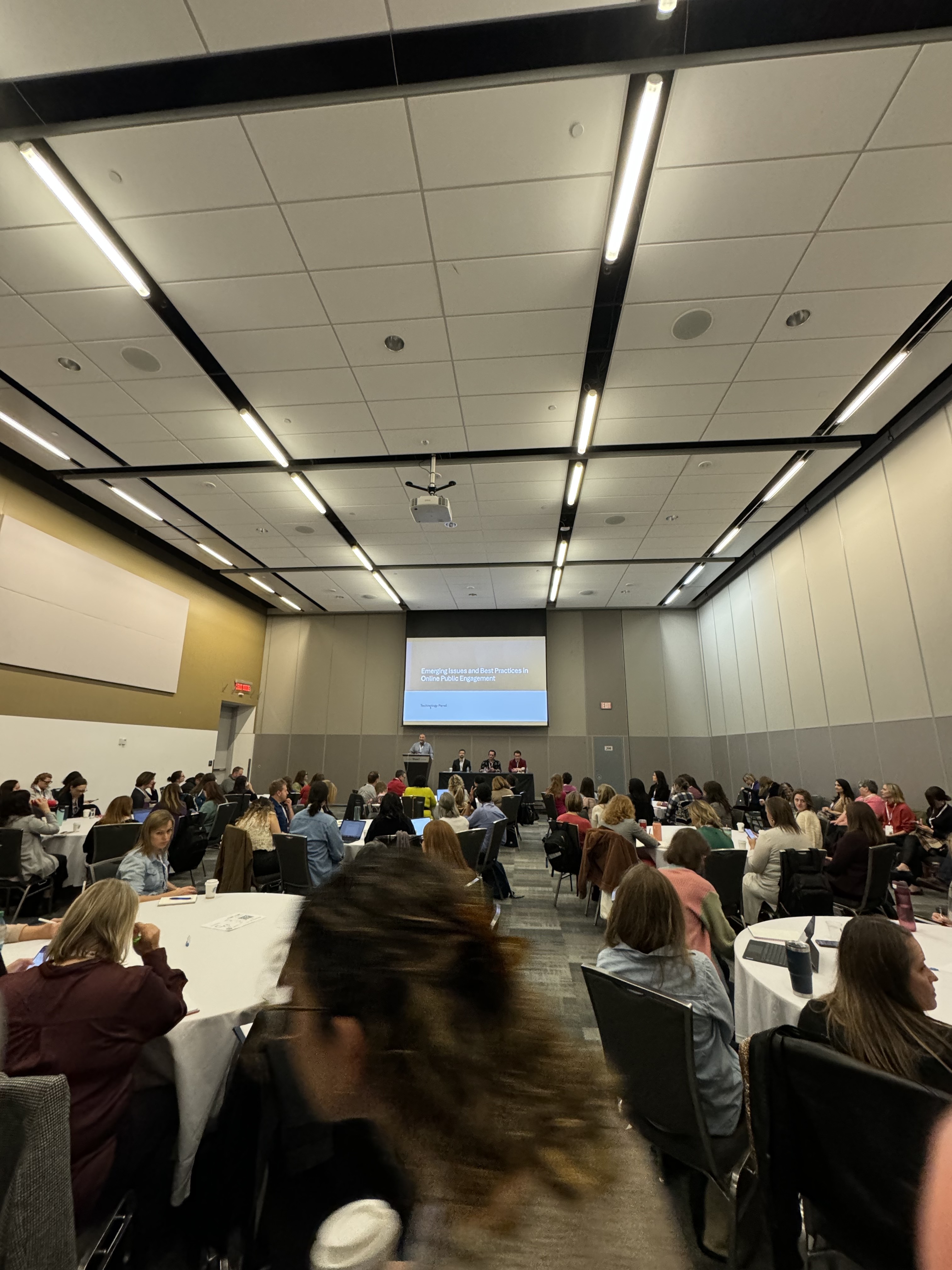
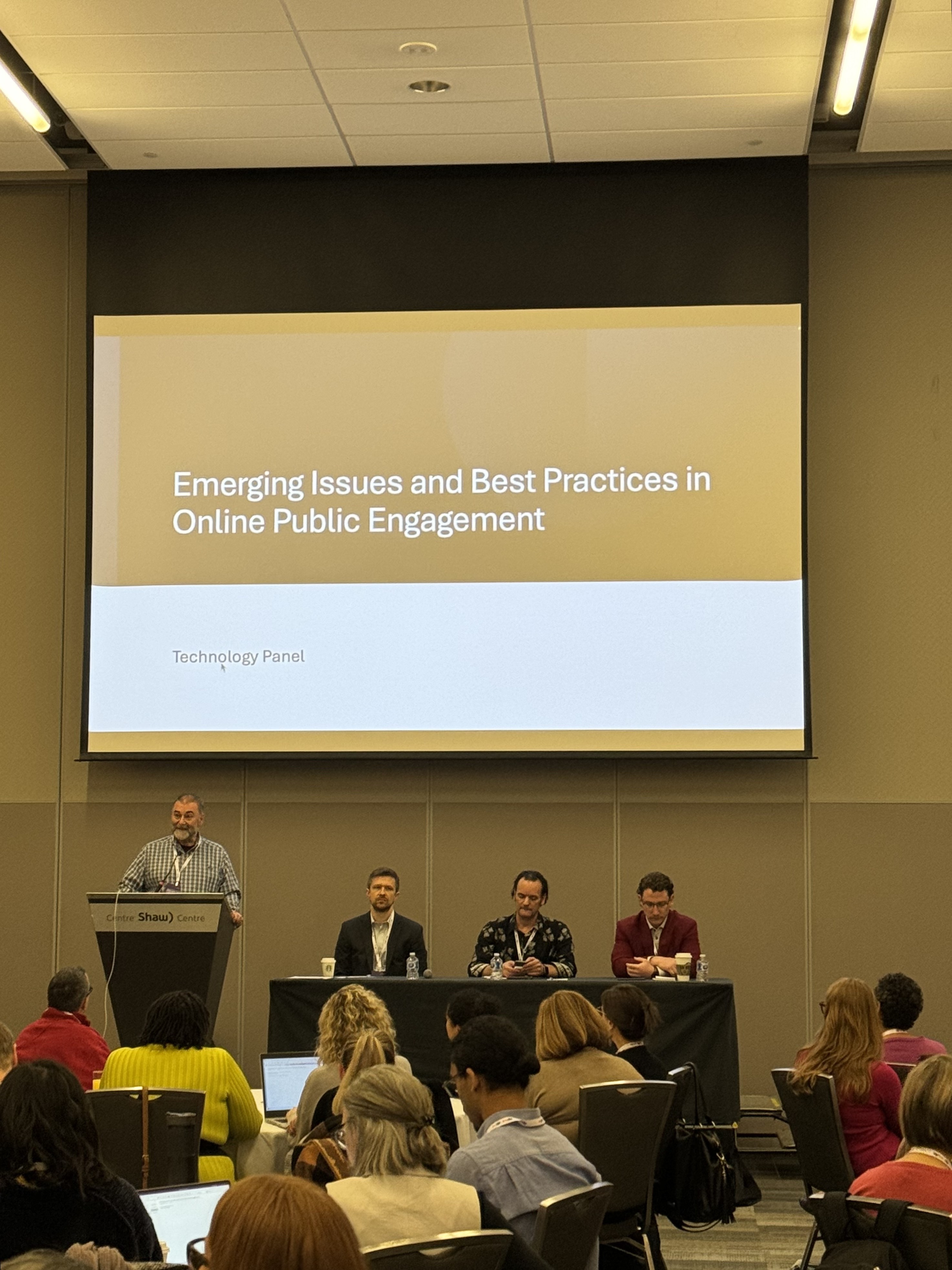
76engage Sponsors the 2024 IAP2 North American Conference in Ottawa
76engage is a proud sponsor of the IAP2 North American Conference taking place this year in Ottawa. The conference brings together thought leaders, practitioners, and experts in the field of public participation to share insights and explore best practices for engaging communities. As a company dedicated to advancing meaningful public engagement, we are excited to support an event that fosters innovation and collaboration across the sector.
At the IAP2 Conference, we look forward to engaging with fellow attendees, hearing from leading public engagement practitioners, and contributing to the ongoing conversations about the future of online and offline engagement. We’re eager to be part of this dynamic community and can’t wait to connect with everyone in Ottawa! Stay tuned for updates on our participation and highlights from the event.

AI and Hybrid Models: Trends in Online Public Engagement to Watch for in 2025
As we move toward 2025, online public engagement is continuing to evolve rapidly. Here are two trends that we anticipate will shape digital public engagement in the year ahead.
Linkworthy
Some articles that caught our attention and that we think are worth sharing.
AI will soon be able to cover public meetings. But should it?
AI may provide a solution to cover meetings that resource-starved news and civic organizations might miss. And it’s important that we develop guidelines with a strong ethical foundation.
Government of Canada Privacy Guidance Checklist
A practical checklist from the Government of Canada of privacy measures to observe in planning, designing, reviewing, launching, and updating a site
Where do we go from X?
Neville Hobson looks at the implications of more fragmented social networks in the wake of Elon Musk’s dismantling of Twitter.
Disney, The New York Times and CNN are among a dozen major media companies blocking access to ChatGPT as they wage a cold war on A.I.
Major news outlets are taking steps to prevent AI applications from scraping their sites to train the AIs’ large language models (LLMs)
Bringing AI to Online Public Participation – Safely and Securely
Since the release of ChatGPT in late 2022, Artificial Intelligence (AI) has exploded in public awareness and everyday use. AI has been introduced in common office productivity apps, email, image generation, search, and online commerce.
But as quickly as the technology is advancing, many words of caution are being raised about establishing norms and guidelines for its use. Technology companies, governments, civil society and users of the technology all have a role in defining the benefits we want to receive and how best to contain the risks.
Public Participation practitioners have begun to experiment with AI tools. And as we use AI, we can see both the promise it holds to enhance meaningful public involvement as well as the risks that it may pose.
76engage is actively exploring the application of artificial intelligence to online public engagement. But as we do this, we are prioritizing the safety of participants and the security of the data you collect, especially participants’ personally identifiable information (PII). We see great potential for AI. But we also understand the risks of doing it wrong. Risks to privacy. Risks to security. Other risks that we may not yet understand?
So, as we start on our AI journey, we have set as a basic policy that AI will be opt-in. Unless you explicitly request that we turn on AI features, they will be turned off. This will give you the control to determine when AI can benefit you and that it fits into your corporate policies.
We also are applying measures to restrict AI from scraping your data from 76engage. Recently ChatGPT published its GPTbot. At the same time they provided a code snippet which we have applied to robots.txt to tell the GPTbot not to scrape content off of a 76engage site. We will look for other opportunities to control access by AI large language models to your content.
As we said, we are experimenting and exploring the possibilities the potential for AI applied in the online space. We have installed some experimental modules and are developing features that use AI to improve the participant experience as well as reporting and analysing their input.
However, to guard your safety and security, we are doing this exclusively on our Lean 76engage site. In this way, we can restrict the AI features to be used only on content that we create for ourselves knowing that it is experimental content and could be shared with the AI models.
We’re very much looking forward to developing these new features. But when we offer them, we want you to be assured that they are both safe and secure for you to use.
So that is our approach, rapid innovation with the safety of your participants in the security of your data as a central goal.
76engage is the Lead Technology Sponsor for the Upcoming IAP2 North American Conference

We are proud to be taking part in the IAP2 North American conference for our fifth year in a row. As a leader in digital engagement and the lead technology sponsor, we will be hosting a breakout session. The session, titled P2 and AI: Promise and Peril, will engage participants in a discussion of the opportunities and the potential issues in using AI for P2. How can AI help with P2? What has it made possible? What are the early issues we are encountering? What strategies, tactics and best practices are we developing to contain the risks while maximizing the benefits of integrating AI into P2?
Along with our breakout session we will also have a booth at the conference. Come find us and say hello. We are eager to learn from, and network with, the top P2 professionals in the industry and come back with new and improved ideas to propel 76engage.
The conference will take place over two and half days in Seattle starting on September 13th. For more information about the conference visit the conference website.
Can’t make it to the conference? Follow the conversation on social media with #iap2nac
IAP2 NAC: Digital Engagement Strategies for a Divided Room
Over 150 people tuned in last month for our IAP2 NAC Technology Panel session: Digital Engagement Strategies for a Divided Room.
Alberta city shows that online engagement is still important for reaching audiences even after the lockdowns
Earlier this week, the City of Brooks Alberta launched their new online public engagement platform. Brooks launched the new platform with their annual budget survey. This new platform, using 76engage, replaces the linear survey they have used in the past and provides an intuitive web experience for community members to engage with their municipal government.
The non-linear design of this years budget survey allows for participants to move through the engagement initiative at their own pace in an order that makes the most sense to them. Instead of working their way through topics they are not interested in, community members can jump right to the departments they care about most. This design structure encourages more meaningful engagement.

The new engagement platform is fully responsive allowing for people to provide input on the go, using smartphones and tablets.
Keep an eye out for more engagement opportunities from the City of Brooks Alberta and to see how they utilize their new engagement platform!
Building Meaningful Digital Engagement with Community: Design and Content Planning
This post is part of a series in which we’ll help you plan, build, promote and manage your online community engagement initiatives. In this post, we’ll explore the design and content planning phases of digital public engagement as well as key considerations for achieving meaningful engagement with community members. We also recommend reading through the first post in this series in which we covered the process of setting goals and reporting objectives for online public engagement initiatives.
In our last post, we discussed how the COVID-19 pandemic reshaped public engagement. As engagement practitioners, the pandemic demanded that we quickly innovate, test and adopt new solutions for all aspects of civic engagement that were no longer possible due to constraints on in-person gatherings; for example, meetings formerly held in community centres moved to virtual town halls and discussions that once took place in-person moved to moderated online forums.
While it appears that we are now at the tail-end of the COVID-19 pandemic as restrictions on gathering have been lifted in most public settings, the advantages gleaned from digital innovation during the pandemic remain. Many organizations are opting to keep their public engagement online to continue reaping the benefits of cost-savings, accessibility, capacity-building, transparency, community ownership and relationship infrastructure that digital public engagement affords. Those that are choosing to return to some level of in-person engagement with community members are considering hybrid strategies that employ both digital and in-person mediums. Thus, even post-pandemic, digital public engagement remains a critical tool in the public engagement toolkit.
In this post, we’ll guide you through several important decision points that you’ll encounter during the design and content planning phases of your next digital public engagement initiative.
Designing the engagement and planning the content
When consulting participants on a particular topic, it can be tempting to duplicate the structure established in a paper or deck that your organization has already prepared on the subject. Instead, identify what the different pieces of content related to the engagement experience will be and consider how you want participants to move between them. Think about how participants interact with the concepts you are engaging them on in the real world. This will likely differ from the highly linear format of publications that are pieces of one-way communication.
The following guidelines come from the field of user experience design. The practice is used extensively in website design and interaction design, and it is intended to help plan experiences that are elegant, intuitive and friendly.
Identifying participants
To create a thoughtful and inclusive engagement experience, it is critical to identify who your participants will be. The characteristics of your participant groups will inform your engagement initiative’s design by directing language requirements, accessibility needs, demands of user effort and other dimensions critical to participation. If you have the resources, identifying your participant personas (characters that represent the different target audiences of the consultation) and stories (goals and motivations) is an excellent practice.
It is important to identify and analyze the full spectrum of your engagement initiative’s targeted participants, paying special attention to underrepresented participant groups who may require additional support to ensure meaningful engagement. The Government of Newfoundland and Labrador’s Public Engagement Guide offers some excellent strategies for accomplishing inclusive engagement with community. The Guide also offers a matrix for mapping consultation stakeholders, which is a useful exercise not only in that it allows designers to identify participant groups but also in that it will yield information about stakeholders’ positions and knowledge levels (The Government of Newfoundland and Labrador, pg 17).
The Government of Newfoundland and Labrador, The Government of Newfoundland and Labrador’s Public Engagement Guide, pg. 17, retrieved from: https://www.gov.nl.ca/pep/files/Public-Engagement-Guide.pdf
Logical grouping of content and activities
Meaningful participation opportunities guide participants to make choices that are informed. As the engagement architect, it is your responsibility to ensure that relevant information and other content are presented to the participant in a logical fashion. Identify what information participants require to make informed choices and identify themes or categories that can be used to organize the different activities. Providing a set of categories can help participants set a mental model that reflects the way they think about the topics in the real world. Organizing information and activities into content groupings will allow you to begin building your engagement initiative’s information architecture. Doing this will not only allow you to ensure that you are not missing any required pieces of context, but also that the right pieces of context are presented to the participant in the right spots. Where possible, it is best to present the participant with the information that is relevant to a particular participation opportunity immediately preceding that opportunity. For example, if your digital engagement with community members asks them about their experiences with the five most commonly sighted birds in North America, it is best to list and describe those five birds immediately preceding the question and input mechanism.
Break it down
Tip: If you are using a discussion paper, break it down into several discussion topics. Present these topics as self-contained spaces or activities and present input prompts in context. Participants will be more likely to provide input if they are not forced to read through a long document, and if they have an easy way to find the topics that are relevant to them.
Linear vs. non-linear structuring
Linear structuring
Your design should help participants move from one activity to another. If the order in which engagement activities are completed matters, then you will need to employ a linear structure that guides participants along a fixed path. This is often the case with surveys that employ screening questions. For example, imagine that you are consulting participants on the climate change mitigation strategies that they would be willing to implement in their neighbourhoods and the list of potential strategies shown to the participant will change depending on what postal code information the participant provides. Since responses to the postal code question will determine the set of strategies shown in the climate change strategies section, this consultation would need to be structured in a linear fashion. Participants would need to be provided with the questions on demographics prior to being provided with the questions on climate change strategies. When constructing linear surveys, consider using pagination to decrease the perceived effort of completing the survey.
Non-linear structuring
More often when conducting digital engagement with community members, you won’t need your participants to complete consultation activities in a particular order. When this is the case, it is best to use a non-linear structure to organize your engagement initiative. Applying a non-linear structure allows participants to complete activities in their preferred order and this often results in higher participation rates because it enables participants to engage on topics that are most important to them first. In a linear consultation, participants may not be shown the topics that they’re interested in until they have completed other activities first; unless a participant is highly motivated to participate, they may lose interest and drop-off, resulting in lower completion rates. When designing a non-linear experience, provide participants with a navigation system that gives users direct access to different activities. It is helpful to offer a completion tracking mechanism (such as 76engage’s activity tracking feature) for participants to see which activities they have completed, and which are pending. Completion tracking can also be used to encourage participants to complete all activities.
When possible, we recommend taking the time to prepare a user flow diagram. This will provide your team with an opportunity to consider your engagement initiative’s requirements for linear vs. non-linear structuring as well as provide a visualization of participants’ journey through the engagement experience.
All at once or phased?
If you need participants to follow a specific flow and if you are consulting over a few weeks or months, consider phasing the publishing of topics. Presenting a few topics at a time can help focus participation and optimize your consultation’s engagement with community members.
Think beyond surveys
Questionnaires are often the first (and in many cases, only) activity that engagement managers consider when planning a digital consultation. However, if you are using a digital engagement platform, then you likely have a range of different activities (such as discussion forums, interactive maps and virtual open houses) available to you that may be better suited to the initiative. Learn about your platform and make use of the features that the digital medium offers. For example, when gathering input about places, such as construction sites, landmarks, or train stations, use interactive maps to give participants a visual way to associate the activities with the places that matter to them most. Additionally, organizations that use a hybrid of in-person and digital mediums to structure their engagement with community members often use interactive maps to plan and display the locations of in-person meetings.
Next up…
Now you have a solid plan: you have identified the objectives, thought through the reporting metrics, and you have chosen the right engagement tools. It is now time to choose the engagement tools and program the activities.
For more information on our state-of-the-art digital engagement platform, visit 76engage.com. Our next blog post looks at choosing the right engagement tools. Stay tuned.
76engage is the Technology Lead Sponsor at the IAP2 North American Conference this year #IAP2NAC
We’re excited to be the Technology Lead Sponsor at this year’s North American Conference in Banff for the International Association of Public Participation. Our CEO, Joseph Thornley, will be presenting a session on Thursday on choice architecture and its impact on online engagement experiences. Be sure to drop by our exhibition booth and let’s discuss how digital engagement can fit into your organization’s engagement strategy.
See you there! #IAP2NAC
Recent Comments